Introduction
In the evolution of value in healthcare, the concept of “value” has become increasingly significant. While the term itself is not new, its meaning within the industry holds a unique and critical importance. In healthcare, value goes beyond the simple cost of implementation and instead focuses on the outcomes and benefits derived from an investment. It spotlights the efficiencies of the system and how they ultimately drive value. In this article, we will explore the multifaceted nature of value in healthcare and its implications for patients, providers, and the overall healthcare ecosystem.
Defining Value in Healthcare
“Value” is a term that is used quite frequently in varying contexts. While not a recent development in healthcare, the term has a unique and critical meaning within the industry. At its most basic core, it speaks to the perceived benefits and the relationship to the returns of the original investment. However, within the healthcare space, value is more focused on outcomes instead of the costs of implementation. This speaks to efficiencies of the system, and how these drive value.
Value-Based Healthcare: Outcomes and Efficiency
Expanding on the above view, value also looks at the outcomes achieved per dollar spent [1]. It plays a crucial role in health economics as it pushes efficiencies and the quality of care provided while considering clinical outcomes and the associated costs. This value-based approach aims for better patient outcomes, improved patient experience, and lower healthcare costs.
There are many aspects to value within the healthcare environment. These look at clinical and cost-effectiveness, as well as patient satisfaction. These areas look at the medical results achieved, the economic outcomes evaluation of the intervention, and the patient’s view or perception of the quality and efficiency of services received.
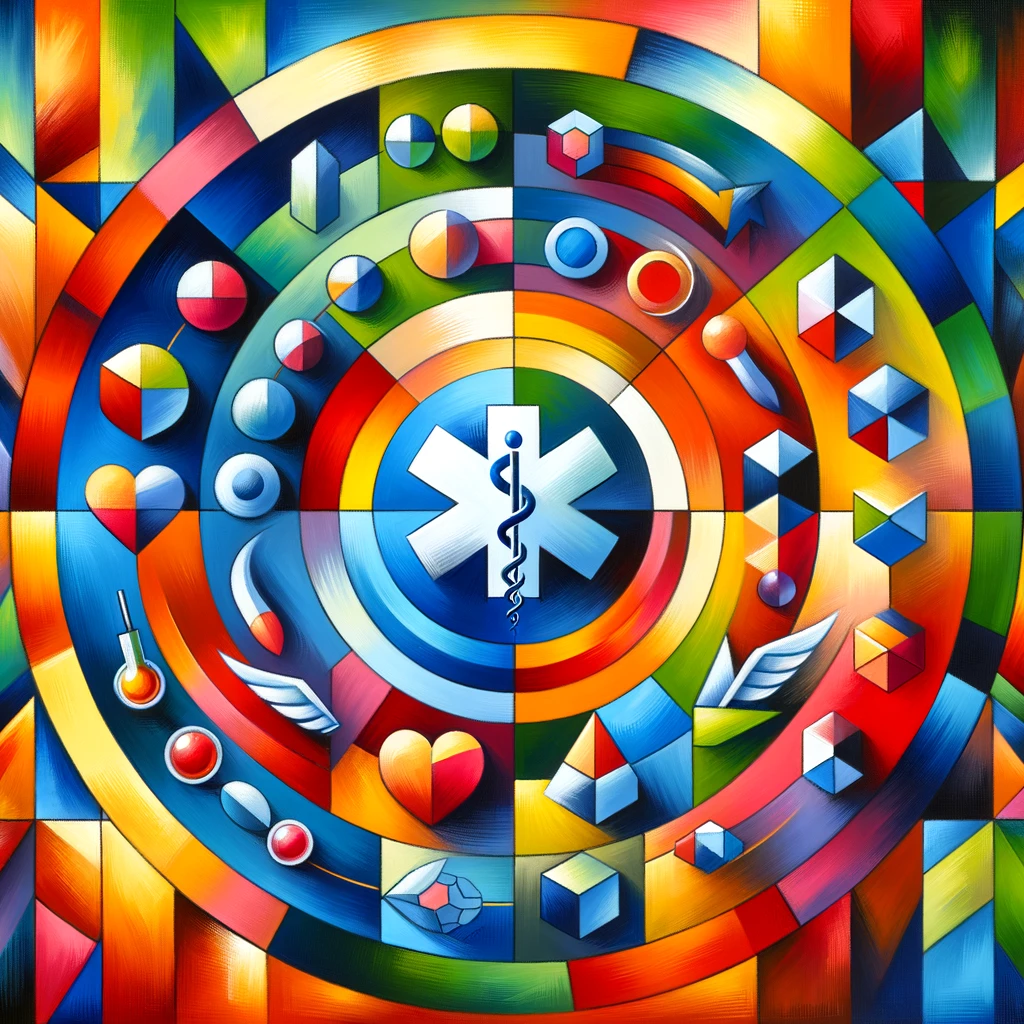
Challenges in Measuring Healthcare Value
However, the evolution of value in healthcare, specifically, measuring value in healthcare presents significant challenges. These interventions can become quite complex as the patient cohort is quite complex in itself. Also, healthcare has to provide a service for a very diverse group of people making outcomes quite difficult to quantify accurately, and the measurement of relative value across diverse groups of people. But despite these difficulties, advancements have been made to create new ways of assessing value. These speak directly to understanding a business case of healthcare – the cost-effectiveness of interventions and their economic outcomes; as well as utilising patients to engage in outcomes reporting (PROMs) [2] .
Value plays a pivotal role in healthcare as costs continue to rise, in a limited-resourced setting, and unlocking efficiencies greatly improves many areas at once – essentially creating a win-win situation. It also encourages healthcare providers to focus on what matters most to patients, leading to more personalised and patient-centred care.
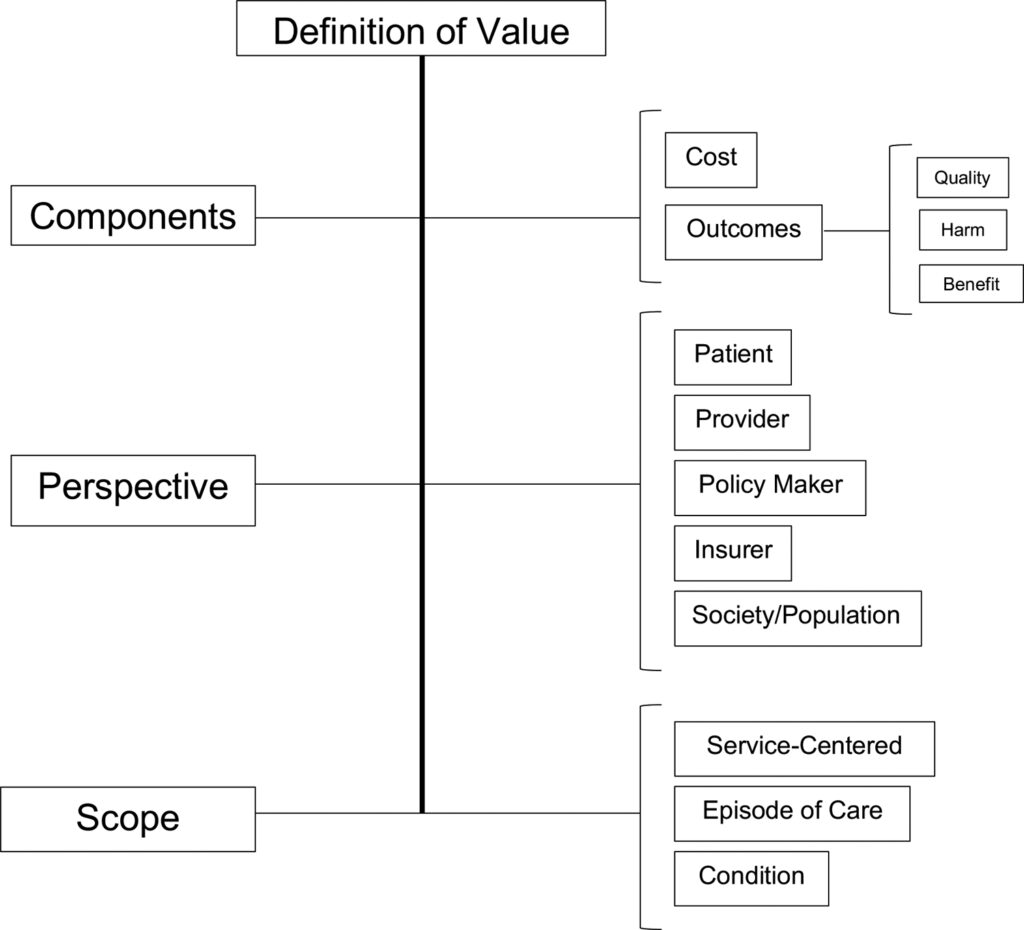
The Role of Artificial Intelligence in Healthcare
Artificial Intelligence (AI) isn’t that new with many examples already showcased. Its utilisation in the healthcare industry, has recently been making big waves due to its impact on providing and designing care approaches. It also can play quite a vital role in improving clinical decision-making by assisting providers with critical information. Of course, the ethical considerations also form significant debates around access and utilisation of AI in applicable industries.
We’ve covered some of these interventions before. But lets quickly review some of these advancements. Machine learning (ML) – which is a subset of AI – is being used to assist radiologists in breast cancer detection [3]. The tool showed that it could match the experts in detecting breast cancer, and in some cases even exceeded them using the scans from mammograms. Similarly, these algorithms have being used to review electrocardiograms (ECGs) and shows the potential of detecting undiagnosed heart conditions [4].
AI and Patient Management
AI then reaches over into the management of conditions that have been identified. By analysing vast amounts of patient data, AI can help doctors predict how a disease will progress and suggest the most effective treatment options. A study showed how AI was used to predict intensive care needs for patients with COVID-19 [5]. Applications such as this contribute to optimising resource allocation efficiencies.
The integration of AI however, is not as easy as it seems. Concerns around data privacy is a major aspect as the systems handle sensitive patient data. With global data privacy regulations, these concerns around confidentiality and security must be addressed. These AI and ML systems need extensive testing to validate their systems, as well as their performance within specific healthcare environments and scenarios. This understanding would provide further evidence as to efficacy within the healthcare settings. AI can often produce varying results depending on what variables are measured, and this still exists within the current picture. This has put a greater emphasis on work in explainable AI to help people understand how it came to the stated outcomes [6] .
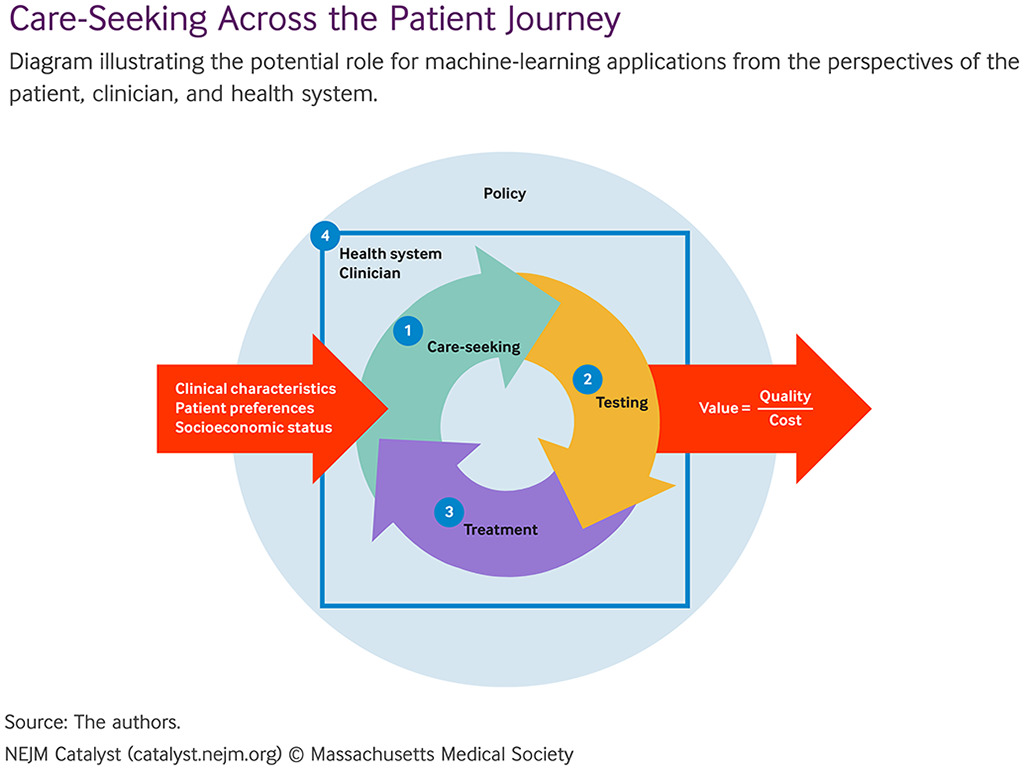
How AI Contributes to Value Creation in Healthcare
The above sections looked to unpack what the basis of “value” is, as well as understanding the “The role of AI”. But we must have a look at how the two areas intertwine. How does AI contribute and create value?
From its most immediate application, AI has the potential to improve efficiencies within the clinical application of healthcare. This is through the role of the provider. There have been numerous reports as well as evidence that show that physician burnout is related to Electronic Health Record utilisation [7] . According to the research, approximately 75% of physicians have indicated this. A manual view of healthcare considers healthcare professionals as managers of every aspect of the healthcare chain. The previous quoted research also indicated that these EHR services were more aligned to billing and documentation instead of healthcare management and patient needs. This speaks to a more value creation view whereby a system takes of care the users, and optimises their processes – both provider and patient.
Optimising Clinical Practice with AI
AI can also act as a fact-checking solution or as a reviewer and assistant for checking the clinical work. This improves the ability to reduce errors. Errors within the healthcare landscape have seen the onset of skyrocketing associated costs as the cascading effects of an error “snowballs”. Ultimately the system can create value by decreasing these errors while ensuring unnecessary interventions and resource utilisation.
AI technologies look to work in these spaces to automate very routine and data-capturing areas of healthcare. This in turn reduces the burden on the providers as well as keeping the patients engaged. We will explore some of these examples in the follow-up issue, but essentially they provide the patients with reminders and prompts based on their current healthcare data. It is this optimisation that creates the various value aspects of healthcare, changing a standard healthcare chain into a value-creation chain. As in, each touch point adds important and crucial information to optimise the service delivery.
The knock-on effect is that of cost saving and decreased workload for the institutions that provide care. Now, while the easiest view from this would be to say it saves hours for more patients to access services, it’s better to look at this time-saving in terms of a cost-waste scenario. So much time is spent through diagnostics and treatment, and this ultimately presents a lower cost efficiency as it speaks to a waste of resources. Hence, this should be viewed as a cost and resources optimisation effort [8] . All of this culminates in early intervention, preventing disease progression and reducing healthcare costs.
Patient experience enhancements are also identified when being provided with a personalised programme. AI systems can monitor health data in real time, provide live feedback, and predict key moments in the healthcare continuum. This also results in higher satisfaction within the healthcare system and increases its subjective value to the person. Ultimately one negative experience is much more damaging than one positive experience. The effects are vastly different. The same actually happens with the clinician. “Loss aversion” refers to the individual placing a higher emphasis on losses than the equivalent gains” [9].
Conclusion
AI has the potential to create value in healthcare by improving clinical outcomes, enhancing patient satisfaction, and reducing costs. Beyond the economic understanding of value driven healthcare outcomes and services, the inclusion of AI into the healthcare industry opens up new opportunities for innovation and improvement. However, we need to unpack this area further to learn of what has been done before and how these have influenced various cost efficiencies within these systems. In the next edition we look to unpack the and explore the economic impact that AI has in healthcare. This will further our understanding of value creation and how to maximise future application of AI for greater value.
References
- Porter, M.E. (2010). What Is Value in Health Care? New England Journal of Medicine, 363(26), pp.2477–2481. doi:https://doi.org/10.1056/nejmp1011024.
- Landon, S., Padikkala, J. and Horwitz, L. (2021). Defining value in health care: a scoping review of the literature. International Journal for Quality in Health Care, 33(4). doi:https://doi.org/10.1093/intqhc/mzab140.
- Awood, M. (2023). AI for Early Identification of Breast Cancer – Efficient and Accurate Diagnosis. [online] Syenza News. Available at: https://news.syenza.com/ai-for-early-identification-of-breast-cancer-efficient-and-accurate-diagnosis/.
- Awood, M. (2023a). AI and ML Enhance ECG Interpretation. [online] Syenza News. Available at: https://news.syenza.com/ai-ml-ecg-interpretation-home-monitoring-cardiac-care/.
- Kim, H.-J., Han, D., Kim, J.-H., Kim, D., Ha, B., Seog, W., Lee, Y.-K., Lim, D., Hong, S.O., Park, M.-J. and Heo, J. (2020). An Easy-to-Use Machine Learning Model to Predict the Prognosis of Patients With COVID-19: Retrospective Cohort Study. Journal of Medical Internet Research, 22(11), p.e24225. doi:https://doi.org/10.2196/24225.
- Loh, H.W., Ooi, C.P., Seoni, S., Barua, P.D., Molinari, F. and Acharya, U.R. (2022). Application of explainable artificial intelligence for healthcare: A systematic review of the last decade (2011–2022). Computer Methods and Programs in Biomedicine, 226, p.107161. doi:https://doi.org/10.1016/j.cmpb.2022.107161.
- Budd J. Burnout Related to Electronic Health Record Use in Primary Care. J Prim Care Community Health. 2023 Jan-Dec;14:21501319231166921. doi: 10.1177/21501319231166921. PMID: 37073905; PMCID: PMC10134123.
- Khanna, N.N., Maindarkar, M.A., Viswanathan, V., Fernandes, J.F.E., Paul, S., Bhagawati, M., Ahluwalia, P., Ruzsa, Z., Sharma, A., Kolluri, R., Singh, I.M., Laird, J.R., Fatemi, M., Alizad, A., Saba, L., Agarwal, V., Sharma, A., Teji, J.S., Al-Maini, M. and Rathore, V. (2022). Economics of Artificial Intelligence in Healthcare: Diagnosis vs. Treatment. Healthcare, [online] 10(12), p.2493. doi:https://doi.org/10.3390/healthcare10122493.
- Hendrix, N., Veenstra, D.L., Cheng, M., Anderson, N.C. and Verguet, S. (2021). Assessing the Economic Value of Clinical Artificial Intelligence: Challenges and Opportunities. Value in Health. doi:https://doi.org/10.1016/j.jval.2021.08.015.
Table of Contents