Introduction
Artificial Intelligence (AI) continues to have a significant impact on healthcare even at its currently steady growth rate. With more and more use cases being developed new tools are emerging to assist with healthcare system strengthening while maintaining and improving value for people who use these systems. This would include both the clinician and the patient as both have specific needs that can be addressed by the AI systems.
It is estimated that the AI in healthcare market growth would increase from US$ 2.1 billion in 2018 to US$ 36.1 billion in 2025, representing a CAGR of 50.2%[1]. With an aging population and the identification of new diseases, an already stressed healthcare workforce is struggling to maintain adequate servicing. In 2013, it was estimated that there was a global shortage of 13 million healthcare workers. This not only decreases the quality of the healthcare services delivered but the increased inefficiencies lead to delayed disease detection and management – again increasing healthcare costs.
The Significance of Early Disease Detection:
Early detection is critical for improving patient outcomes and reducing healthcare costs. In studies performed in cancer populations, it was found that costs increase with each higher stage, with later cancers having costs 7 times higher [2]. Sepsis was noted as one of the leading causes of death in US hospitals. Each year, 1.7 million adults in the US develop sepsis, and unfortunately, 1 in 3 people die from it during their admission [3]. The current diagnostic methods require lengthy testing which wastes precious time [4], not to mention the increased costs of hospital utilisation during these events.
The curative model sits on the basis of outputs that guide healthcare professionals on the most accurate and effective way to provide treatment. While many providers would argue that experience and the “gut feel” of the correct path allow them to speed up this process, any decision that’s not made based on evidence could be detrimental to the person and could start a downward spiral of poor outcomes, high cost, and more importantly a poor experience for the person going through this event. There are conditions that exist where until a specific symptom has appeared, or is experienced, the diagnosis and condition remain far from being identified.
Managing Healthcare Costs
Healthcare is associated with many avenues to an outcome, which relate to not only testing for peace of mind and ruling out serious diagnoses but also being efficient and waiting until the “right” time and using the “right” test. Unfortunately, very open and loose rules such as these could result in poor efficiencies, and therefore poor experiences. A systematic review in 2022 showed the substantial overuse of testing especially in the areas of preoperative testing and imaging [5]. The overuse of testing also does not increase the quality of care, in some cases its quite the opposite with recurring visits and personal discomfort. Increased utilisation of testing can also increase the chance of incorrectly identifying medical problems with data showing that around 5% of healthy people receiving abnormal test results [6].
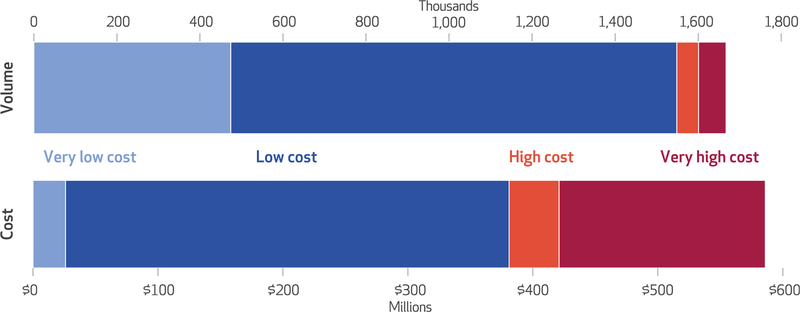
The US healthcare costs have a larger spend in low-value care where the overall benefit of this is poor [7]. Even with access through low cost assessments that could drive the early identification process, the higher utilisation presents another problem within the system – value. Value needs its own breakdown, something we’ll do in the coming weeks, but value relies on efficiencies for both the person accessing, and the provider performing, the treatment. Increasing costs on either side decreases value [8]. Fundamentally then, the question becomes how can a system be optimised for providing early detection to increase which leads to cost efficiencies. This is where AI has shown great potential and impact.
Artificial Intelligence in Early Disease Detection
The advancements in AI and healthcare have seen many leaps with AI showcasing a growing number of use cases – especially in early disease detection [9]. Instead of creating new data points on people accessing care, some of the initial efficiencies have been found in existing data. Using advanced machine learning which can access the plethora of medical data lakes, diseases and diagnoses are being identified. In some cases, diagnoses aren’t put forward, however, a risk index is presented indicating that a problem might exist or is developing.
Early models of this detection work to understand the population dynamics and incidence. Using this as a guide, a clinical decision could be made given the inference on the population’s needs. At a macro level, exposomes provide a view of the environment. Understanding its chemical, dietary, and social factors can highlight critical biomarkers linked to disease. Another layer added to this is screening, however, this is not without its own difficulties noted above. Lead-time bias is prevalent as a waiting time exists without changing the ultimate outcome [10]. This is an area where AI can see great traction and influence as it looks to decrease and minimise this waiting time, and to provide an outcome in advance.
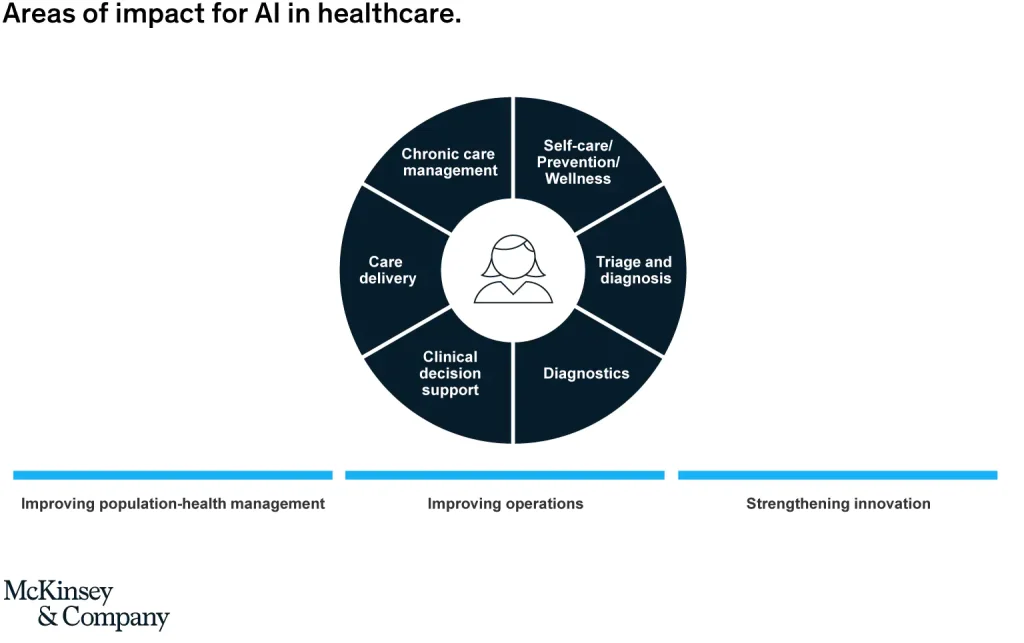
AI has been used in various cancer-related screenings and detection. Multi-Cancer Early Detection (MCED) has seen increased utilisation in recent years as it offers a means to detect various types of cancers early. Research indicates that adding a MCED test in standard care enabled the early identification of cancers and led to a reduction of “late-stage incidence” by 78%. This in turn has the potential to decrease 5 year cancer mortality by 39% [11].
Some early detection AI work has taken this a step further by looking at real-time or live data. A biocompatible AI chip was produced to monitor heartbeats. This chip could accurately differentiate between three common arrhythmias with 88% accuracy [12]. This allows for close monitoring and prediction of any adverse effects in real-time.
Deep learning models, and algorithms such as COMPOSER (COnformal Multidimensional Prediction Of SEpsis Risk) and InSight, look into understanding and predicting sepsis risks. COMPOSER looks to reduce false alarms due to poor data and would flag the respective cases. Importantly it provided an improved early warning which ensured appropriate prioritisation of those that required it. InSight uses six vital signs to predict the different types of sepsis, where it outperformed existing systems [13]
Remote patient monitoring and early detection
Biofourmis is a remote patient monitoring company in the US that makes use of advanced AI to monitor and predict the risk classification of the person using the system. Their Biovitals™ Analytics Engine received FDA approval in 2019 [14] and is used in research and remote patient monitoring. While their work focuses more on the early discharge and de-escalation of care, with a shift to the home environment (transitional care), the AI monitors the person’s vital signs and generates clinically relevant insights. The system provides features such as autonomous monitoring, with alerts being provided to clinicians as relevant and needed. These alerts not only allow the clinician to shift their focus to another task, but they also provide predictive analytics to early identify those whose risk classification is escalating – saving valuable time. The system was able to predict heart failure decompensation 12 days before the admission. The system understood the multiple comorbidities of the person and could inform the team of the various next steps.
While this is in the remote patient monitoring space, it demonstrates the power of early detection and AI. The example highlights efficiencies that can come about through the optimisation of biomarker identification. The clinician is able to provide more clinical input through their continuum. For the person, early identification analytics enables them to be constantly monitored whilst receiving back-end support. The healthcare system benefits because not only does this provide higher cost efficiencies, but also autonomy in health for both person and provider. The cost reduction is experienced for all parties due to lower utilisation of critical healthcare resources – this would be felt by the patient too, but it also opens the door for optimised management of the doctor resource leading to enhanced remuneration and resource planning.
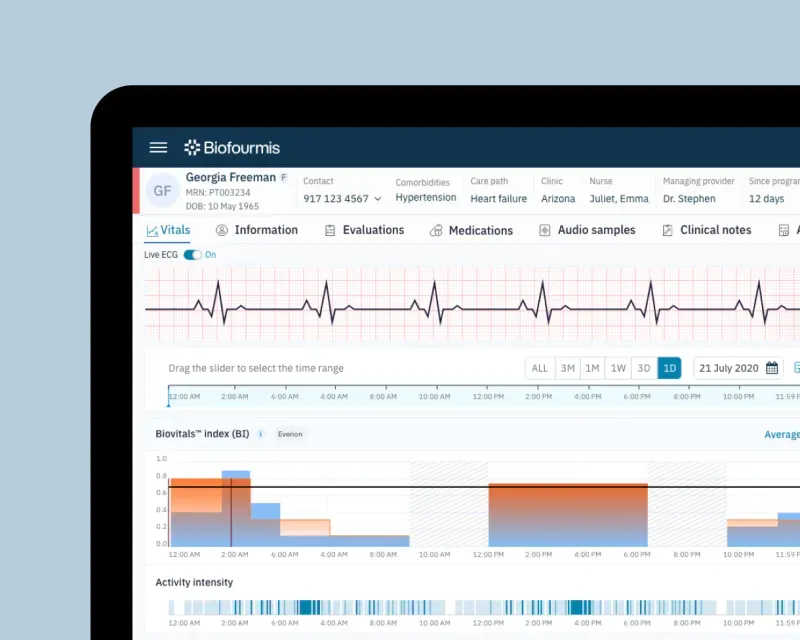
Conclusion
The need for early disease detection has been well established. There is the benefit experienced on the service level provision, however, on the personal experience it has been widely underutilised. The pockets where it does exist detail the greater provision for healthcare services – improving access and outcomes. AI takes this even further by applying a personalised approach to care, specific to the person that needs it. This just-in-time approach looks to curb wasteful expenditure which ultimately provides little to no value to the final outcome. AI improvements of late have also demonstrated its improved image recognition capabilities, as well as enhanced natural language processing and data extraction while “reading” transcripts, EHR data, or monitoring a conversation.
In leveraging and enhancing the technology, better systems can be deployed ensuring that a healthcare provider has the most up-to-date information but also information that reflects a person’s real-time risk thereby prompting further action. This has been demonstrated in pre-clinical need cases as well as post-acute settings. There is still further value to be unlocked as these systems roll out into the greater public domain.
References
- Hazarika I. Artificial intelligence: opportunities and implications for the health workforce. International Health. 2020;12(4). doi:https://doi.org/10.1093/inthealth/ihaa007
- Reddy SR, Broder MS, Chang E, Paydar C, Chung KC, Kansal AR. Cost of cancer management by stage at diagnosis among Medicare beneficiaries. Curr Med Res Opin. 2022 Aug;38(8):1285-1294. doi: 10.1080/03007995.2022.2047536. Epub 2022 Apr 20. PMID: 35285354.
- Centers for Disease Control and Prevention. What is sepsis? Centers for Disease Control and Prevention. Published 2022. https://www.cdc.gov/sepsis/what-is-sepsis.html
- Desautels T, Calvert J, Hoffman J, Jay M, Kerem Y, Shieh L, Shimabukuro D, Chettipally U, Feldman MD, Barton C, Wales DJ, Das R Prediction of Sepsis in the Intensive Care Unit With Minimal Electronic Health Record Data: A Machine Learning Approach JMIR Med Inform 2016;4(3):e28 doi: 10.2196/medinform.5909 PMID: 27694098 PMCID: 5065680
- Müskens JLJM, Kool RB, van Dulmen SA, Westert GP. Overuse of diagnostic testing in healthcare: a systematic review. BMJ Qual Saf. 2022 Jan;31(1):54-63. doi: 10.1136/bmjqs-2020-012576. Epub 2021 May 10. PMID: 33972387; PMCID: PMC8685650.
- C. Bruce Alexander, Message from the President: Reducing Healthcare Costs through Appropriate Test Utilization, Critical Values, Volume 5, Issue 2, 1 April 2012, Pages 6–9, https://doi.org/10.1093/criticalvalues/5.2.6
- Mafi JN, Russell K, Bortz BA, Dachary M, Hazel WA Jr, Fendrick AM. Low-Cost, High-Volume Health Services Contribute The Most To Unnecessary Health Spending. Health Aff (Millwood). 2017 Oct 1;36(10):1701-1704. doi: 10.1377/hlthaff.2017.0385. PMID: 28971913; PMCID: PMC6727655.
- Ernst R. Indirect Costs and Cost-Effectiveness Analysis. Value in Health. 2006;9(4):253-261. doi:https://doi.org/10.1111/j.1524-4733.2006.00114.x
- Spatharou A, Hieronimus S, Jenkins J. Transforming healthcare with AI: The impact on the workforce and organizations | McKinsey. www.mckinsey.com. Published March 10, 2020. https://www.mckinsey.com/industries/healthcare/our-insights/transforming-healthcare-with-ai
- Schiffman JD, Fisher PG, Gibbs P. Early Detection of Cancer: Past, Present, and Future. American Society of Clinical Oncology Educational Book. 2015;35(35):57-65. doi:https://doi.org/10.14694/edbook_am.2015.35.57
- Earl Hubbell, Christina A. Clarke, Alexander M. Aravanis, Christine D. Berg; Modeled Reductions in Late-stage Cancer with a Multi-Cancer Early Detection Test. Cancer Epidemiol Biomarkers Prev 1 March 2021; 30 (3): 460–468. https://doi.org/10.1158/1055-9965.EPI-20-1134
- Technische Universität Dresden. “Using artificial intelligence for early detection and treatment of illnesses.” ScienceDaily. ScienceDaily, 20 August 2021. https://www.sciencedaily.com/releases/2021/08/210820135346.htm
- Zhang D, Yin C, Hunold KM, Jiang X, Caterino JM, Zhang P. An interpretable deep-learning model for early prediction of sepsis in the emergency department. Patterns. 2021;2(2):100196. doi:https://doi.org/10.1016/j.patter.2020.100196
- Biofourmis. Biofourmis Earns FDA’s First-Ever Breakthrough Device Designation for a Novel Digital Therapeutic for Heart Failure. www.prnewswire.com. Published July 29, 2021. Accessed September 11, 2023. https://www.prnewswire.com/news-releases/biofourmis-earns-fdas-first-ever-breakthrough-device-designation-for-a-novel-digital-therapeutic-for-heart-failure-301344019.html
Table of Contents