Transforming Mental Health Care Access and Delivery
Before COVID-19, telemedicine was already revolutionising the healthcare industry. Research in the United States has shown that 61% of healthcare institutions made use of some type of telehealth. [1] It provided a convenient platform for patients to access healthcare services remotely, reducing the need for physical visits to healthcare facilities. It not only improves access to medical services for people living in remote areas but also for those with limited mobility or transportation. Telemedicine also facilitated better management of chronic diseases and made healthcare more cost-effective. However, despite these advantages, the full potential of telemedicine (and Artificial Intelligence-enabled Mental Health Care, discussed below) was yet to be realised before the pandemic hit. Since then, a plethora of technologies have emerged, and the golden age of artificial intelligence began allowing many industries to flourish – and healthcare was no exception.
Healthcare adoption was quick in seeking optimisation and efficiency in a time of low contact, as it took on various prioritisation efforts to provide care to those who urgently needed it. With health systems seeing higher usage and shift towards a digital medium, the top desired outcomes of a digital transformation were satisfaction and engagement (92%), and secondly improved quality of care (56%). [2] Downstream efficiencies were realised and service augmentation began. Providers who looked to innovate and digitise their practices saw enhanced business practices, and patients could participate in their care programs at a similar or better level through a digital medium. Outcomes were maintained – or better – and a wider net of access and support was created. In some examples, low-acuity visits that were performed online resulted in higher efficiencies in time and cost; and they showed a decrease in physical follow-ups (around 13.5%), with less than 1% of people using the emergency services. [3]
Not only were the benefits of AI in healthcare realised in servicing healthcare needs, but they were also found in the administration of the services. Prime Therapeutics – a Pharmacy Benefit Manager, used its advanced fraud, waste and abuse (FWA) program to save $285 million for the period 2020 to 2021. Their processes first look through the various clinical programs and formulary to identify some of the FWA. It analyses these clinical products (pharmacy and medical data) and then filters them through the core FWA product which focuses on pharmacy risk mitigation services. Finally, it is then analysed by their award winning Advanced FWA which ranks risks by a risk rating to identify outliers based on predictive modeling. The program helped investigators identify an increase of 60% in false prescription drug claims. [4]
Specialised healthcare services, such as mental health, looked to the innovations in telemedicine that are paving the way for more accurate and personalised diagnostics. The unmet need was recognised during the COVID-19 pandemic for mental healthcare services and despite its growing importance, significant challenges have hindered its widespread and effective utilisation. Virginia Commonwealth University (VCU) Health System has been offering telepsychiatry since 1995, but there was very little usage. However, after COVID-19, this changed dramatically, with clinical volume growing to 120%. [5] Stigma and limited access to timely assessments have often resulted in underdiagnosis and delayed intervention with recent research suggesting 65% of individuals with mental health disorders do not seek professional help due to this. The intersection of AI and healthcare presents opportunities to bridge this gap. Groundbreaking approaches in Artificial Intelligence-enabled Mental Health Care such as AI-driven voice analysis and chatbots hold the potential to transform how mental health disorders are identified and treated, offering hope for overcoming these longstanding obstacles.
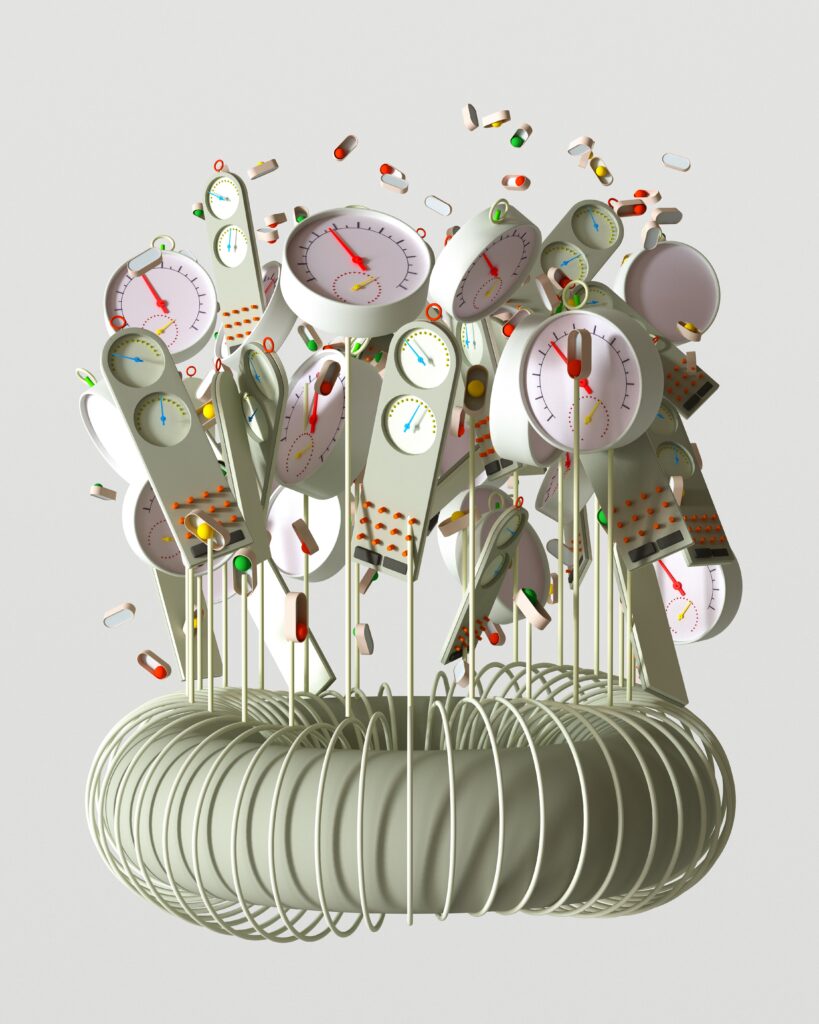
Artificial Intelligence’s Role in Improving Mental Health Care
Revolutionising mental health detection and patient engagement
AI has revolutionised the field of mental health by facilitating early detection of symptoms. This is done through constant interaction within the application or system. Machine learning algorithms can analyse patterns in speech, and text to predict the onset of conditions like depression and anxiety. These have seen increased proliferation in the market due to an increasing number of people who are opting for AI services in healthcare over traditional face-to-face options. This is supported by a report published by Accenture which states that 46% of respondents would prefer to receive their mental healthcare services online. [6]
This insight presents the comfortability in the usage of these systems, and it also nudges one to think about the experience of sharing in these situations. These systems have quickly become anthropomorphic and much more relatable allowing people to fully engage with them and provide support at any given moment. Evidence backs this up showing that telehealth performs the same if not better than in-person treatment.[7] For example, apps that provide AI-powered chatbot therapy, offer immediate emotional support and coping mechanisms. These advancements have significant implications for mental health care, enabling timely intervention and potentially preventing the escalation of mental health conditions.
The provider also gains enhanced insights into their patients’ engagement with a platform. NLP (Natural Language Processing) is a method of understanding the spoken or written word. ieso – the online mental health clinic uses this technology to provide these technologies to improve the interventions that patients would receive. Data gathering is an intensive exercise, but by leveraging deep learning models they could better understand how clinical outcomes were influenced by patient language and their interventions. [8]
Machines are then able to link various data points from this – and with other data sources such as EHRs (Electronic Health Records) to offer a unique way forward for the patient. There is currently also the development of quality controls through using these techniques.
Artificial Intelligence-powered personalised treatment strategies
Early detection of mental health symptoms through AI apps and machine learning provides a proactive approach, potentially identifying issues before they become severe. Added to this, the added value presented is unlocking personalised treatment plans based on the person’s unique patterns and symptoms. It improves accessibility, allowing those who may not have easy access to traditional mental health resources. With continuous monitoring and providing real-time data to healthcare providers, better decision-making in patient management occurs. Furthermore, it reduces stigma around seeking help for mental health issues, as individuals can start their mental health journey in private.
Patients benefit from this personalised care through improved treatment efficacy and reduced side effects. It also empowers patients to take an active role in their care, enhancing their treatment adherence. Apps like Woebot, and Ginger.io, use machine learning to understand what it is that the person needs based on their interactions. In a clinical trial, participants using the Woebot application were found to have their symptoms of depression “significantly reduced” when compared to participants who only used information developed and provided by the NIH. [9] Ginger.io also makes use of machine learning, but its approach is to suggest the correct level of care – provided in the app – as needed by the patient irrespective of time or place.
For providers, AI-assisted personalisation improves patient management by streamlining care processes and reducing the burden of manual data analysis. It also assists in decision-making, providing evidence-based recommendations for treatment adjustments.
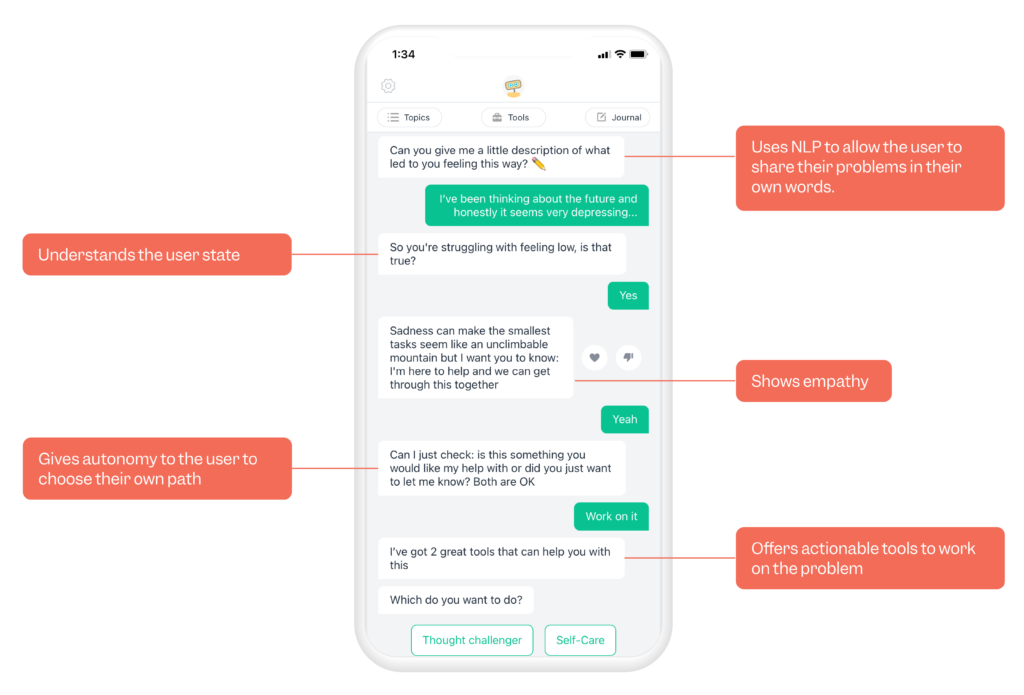
Navigating biases and data security
Algorithms and various other organised forms of data detailing and prediction are built by humans, and it has been well documented that existing biases (such as racial, gender, sexuality, and age) could cross over into these applications or systems. [10][11][12] This is called “algorithmic bias”, and it can continue to spur on current societal problems such as discrimination, privilege, and inaccessibility that have existed before. These would still proliferate even without any human interaction.
Data security has come under much scrutiny post COVID-19 due to the shift in digital health technologies providing care services. Many apps are available for download however, they do not comply with the various data privacy regulations of a given country. Recently apps have been banned in some countries, with others having to pay fines to the Federal Trade Commission (FTC) for sharing sensitive data with advertisers. It is suggested that developers or platform providers take the initiative to enhance privacy. [13] From a healthcare provider’s perspective, AI requires a level of risk and trust management as it would provide them with potential diagnoses or identify biomarkers of concern. When acting on these data points, questions of autonomy and liability are inevitable. [14] From the person’s perspective, the risks associated with this level of decision-making could lead to a myriad of down-the-line ramifications.
Conclusion
The synergy between AI and healthcare is transforming mental healthcare, offering potential solutions to longstanding challenges. Physical and psychosocial barriers can both be addressed through the use of technology. This unlocks value by improving treatment strategies and enhancing the care experience through early detection and personalizing treatment goals. As this space continues to evolve, issues regarding data privacy, bias, and decision-making must be addressed to ensure the responsible and effective use of AI-based solutions.
Key takeaways:
- Telemedicine was already transforming the healthcare industry before COVID-19, improving access for people in remote areas or with limited mobility and making healthcare more cost-effective and convenient.
- AI and telemedicine have significant potential within mental health that could enable better decision-making and treatment, particularly as stigma and limited access often result in underdiagnosis and delayed intervention. It has facilitated early detection of mental health symptoms through analysis of speech and text, with apps providing immediate support and ongoing therapy. AI also provides personalised treatment strategies and empowers patients to take an active role in their care.
- Ethical considerations remain a high priority. Existing biases could cross over into applications; concerns around data security and privacy have increased; and issues of autonomy and liability from both the healthcare provider’s and patient’s perspective remain unanswered.
References
- Luna, Paulina, et al. “Telehealth Care before and during COVID-19: Trends and Quality in a Large Health System.” JAMIA Open, vol. 5, no. 4, 4 Oct. 2022, www.ncbi.nlm.nih.gov/pmc/articles/PMC9531686/, https://doi.org/10.1093/jamiaopen/ooac079. Accessed 31 Oct. 2022.
- Appleby, C., Hendricks, J., Wurz, J. and Shudes, C. (2021). Digital transformation. [online] Deloitte Insights. Available at: https://www2.deloitte.com/us/en/insights/industry/health-care/digital-transformation-in-healthcare.html.
- Shaver, Julia. “The State of Telehealth before and after the COVID-19 Pandemic.” Primary Care: Clinics in Office Practice, vol. 49, no. 4, Apr. 2022, www.ncbi.nlm.nih.gov/pmc/articles/PMC9035352/, https://doi.org/10.1016/j.pop.2022.04.002.
- LLC, Prime Therapeutics. “Prime Therapeutics’ Advanced Fraud, Waste and Abuse Program Saved Its Health Plan Clients $285 Million in 2020.” Www.prnewswire.com, 11 Aug. 2021, www.prnewswire.com/news-releases/prime-therapeutics-advanced-fraud-waste-and-abuse-program-saved-its-health-plan-clients-285-million-in-2020-301352999.html
- Strazewski, Len. “Uncovering the True Value of Telehealth to Improve Care Outcomes.” American Medical Association, 18 Oct. 2021, www.ama-assn.org/practice-management/digital/uncovering-true-value-telehealth-improve-care-outcomes.
- Accenture (2020). How Can Leaders Make Recent Digital Health Gains Last? https://www.accenture.com/_acnmedia/PDF-130/Accenture-2020-Digital-Health-Consumer-Survey-US.pdf#zoom=40
- Hubley S, Lynch SB, Schneck C, Thomas M, Shore J. Review of key telepsychiatry outcomes. World J Psychiatry. 2016 Jun 22;6(2):269-82. doi: 10.5498/wjp.v6.i2.269. PMID: 27354970; PMCID: PMC4919267.
- Ewbank, M. P., et al. “Understanding the Relationship between Patient Language and Outcomes in Internet-Enabled Cognitive Behavioural Therapy: A Deep Learning Approach to Automatic Coding of Session Transcripts.” Psychotherapy Research, vol. 31, no. 3, 3 July 2020, pp. 300–312, https://doi.org/10.1080/10503307.2020.1788740
- Fitzpatrick K, Darcy A, Vierhile M Delivering Cognitive Behavior Therapy to Young Adults With Symptoms of Depression and Anxiety Using a Fully Automated Conversational Agent (Woebot): A Randomized Controlled Trial JMIR Ment Health 2017;4(2):e19 URL: https://mental.jmir.org/2017/2/e19 DOI: 10.2196/mental.7785
- Fiske A, Henningsen P, Buyx A Your Robot Therapist Will See You Now: Ethical Implications of Embodied Artificial Intelligence in Psychiatry, Psychology, and Psychotherapy J Med Internet Res 2019;21(5):e13216 URL: https://www.jmir.org/2019/5/e13216 DOI: 10.2196/13216
- Ziad Obermeyer et al. ,Dissecting racial bias in an algorithm used to manage the health of populations.Science366,447-453(2019).DOI:10.1126/science.aax2342
- Straw I, Callison-Burch C. Artificial Intelligence in mental health and the biases of language based models. PLoS One. 2020 Dec 17;15(12):e0240376. doi: 10.1371/journal.pone.0240376. PMID: 33332380; PMCID: PMC7745984.
- Iwaya, L.H., Babar, M.A., Rashid, A. et al. On the privacy of mental health apps. Empir Software Eng 28, 2 (2023). https://doi.org/10.1007/s10664-022-10236-0
- Naik N, Hameed BMZ, Shetty DK, et al. Legal and Ethical Consideration in Artificial Intelligence in Healthcare: Who Takes Responsibility? Frontiers in Surgery. 2022;9(862322):862322. doi:https://doi.org/10.3389/fsurg.2022.862322
Table of Contents