Introduction
Navigating Artificial Intelligence (AI) in Healthcare Deployment has brought many benefits and challenges. Integrating it into the healthcare sector will still be a difficult task. Concerns about transparency, ethics, and justice, as well as computer biases and privacy – all of which can have lasting effects on healthcare if they are not addressed appropriately.
Large organisations and regulatory bodies have attempted to help, by providing insights into best practices for AI-based clinical application. To this end, six guiding principles have been identified: protecting autonomy, supporting human well-being, transparency, encouraging responsibility, encouraging inclusivity, and promoting responsive and sustainable AI. [1] However, even with these principles, it has been hard to keep up with the rapid growth of AI. It continues to grow, and continues to expand its grasp. While the improvements have been seen, its even more critical now that we understand the ethical implications that could be presented. This article looks to understand these challenges and to propose a framework for evaluating AI tools before their deployment.
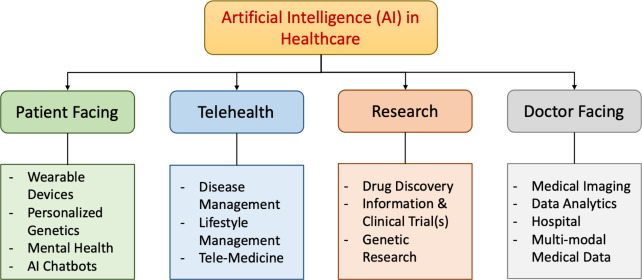
Current Ethical Landscape for Artificial Intelligence
Healthcare companies have been investing a considerable amount of resources into AI capabilities. However, glaring gaps within the “ethical fibre safety net” have been used as risk factors when implementing these systems. Because of these ever-growing investments, its then understandable that AI’s rapid deployment has allowed poor scrutiny of crucial ethical structures.
AI was quoted as “Augmenting Inequality” [2] during the COVID-19 pandemic. The benefits of AI were overshadowed by the reports of systems creating poor outcomes within sociodemographic groups. The disproportional damage resulted in a compounding effect on the disadvantaged groups during COVID-19. Data is typically in silos which allows for crucial access control protocols. While this is a good way to protect information, it allows for the “built-in” criteria – used to define the data – to continue exhibiting the same tendencies and trends. This could essentially perpetuate what is an already skewed view.
This then poses the question: “Why was this data needed?” Within any process, optimisation attempts are constantly being evaluated so as to optimise and improve the value chain. Within healthcare, this is no different. Naturally, more data points provided leads to increased predictiveness and likelihood, but it’s usually through the overprescription of data points that some of these ethical dilemmas manifest themselves. These AI deployments are thought of as designed for value. [3] We see this through many daily activities. An easy example of this is advertisements. The AI or robots initially provided people with “click-bait” to create value or revenue for the creator. However, the person following the action would then be met with further clicks to go back to their original activity, and if they don’t, they were usually met with a payment for something they did not know they wanted – let alone needed. What is the missing link here? Human-centric design. Before we can unlock the value chain, the question of the intention or purpose of the proposed solution must be answered first. This can only be done using ethically sound principles.
Considerations for Artificial Intelligence Implementation
There are numerous aspects that need careful consideration and review. These include ethical AI, explainable or interpretable AI, health equity and algorithmic biases, sponsorship bias in medical literature, data privacy, genomics and privacy protection, and issues related to sample size and statistical significance. [1]
Ethical Artificial Intelligence
Ethical AI in clinical practice requires a greater emphasis on the areas of informed consent, patient privacy, and the role of physicians in AI-mediated care. Important factors to include are ethical guidelines, transparency, patient confidentiality, and stakeholder engagement. AI is already using patient data to improve healthcare decision-making. This is provided in the form of Electronic Health Records (EHRs) and Generative AI clinical assistants. The main goal is to share real-time data to make it easier to assist in clinical decisions such as diagnostics and improving outcomes. [4]
Explainable Artificial Intelligence
Explainable AI (XAI) is an important area in healthcare as it looks to explain the AI process. AI is a high-stakes area as it’s still seen as a bit of a black box [5] due to the limited understanding or knowledge as to how the algorithm was created. This growing field of research is important as it looks to understand ML machine learning (ML) and to ensure its reliability. In turn, this would allow for better explainability and interpretability, thus ensuring a tool the person can trust, is user-friendly, and fit for purpose. Efforts should be made to enhance AI’s explainability and interpretability, ensuring that AI tools are trustworthy, user-friendly, and fit for purpose.
Health Equity and Algorithmic Biases
Health equity and algorithmic biases in AI possess powerful effects in inequalities in healthcare. They can increase these gaps. AI, unfortunately still retains its flawed inherent biases. Research has shown examples of facial recognition software misidentifying people of colour more often which leads to wrongful arrests. [6]Other examples include poor detection of people of colour in self-driving cars [7] and poor detection of speech recognition. [8] Interestingly and often understated are the reinforcements of gender bias through the use of virtual assistants with submissive traits.[9] Efforts should be made to eliminate these biases at every stage of AI system development.
Sponsorship Bias
Sponsorship bias has been detailed many times in medical literature. These were shown where sponsored studies tend to produce results favoring the sponsor, raising concerns about the integrity of clinical studies. While not particularly new in the literature, it however plays a critical role in the outcomes of trials, and other research efforts. This has to do with the publication bias which threatens credibility. This was a usual occurrence in the early days of clinical trials when the main goal was to make sure that a drug was approved – specifically to ensure licensing drugs – and to profit off of them. [10]
Data Privacy
Data privacy is becoming a more critical aspect of healthcare, especially with the popularity of AI technologies like facial recognition and AI chatbots. These multimodal applications have become commonplace in healthcare. Natural Language Processing (NLP) and face recognition is being used to ensure a level of security. [11] Cybercrimes have increased in this digital age of healthcare. Recent statistics share that the largest data breach in healthcare (in the US) affected 78.8 million individuals, with the average cost of a healthcare data breach equaling 10.93 million USD. [12]
Genomics and Privacy Protection
When it comes to genomic research, privacy and consent models face many challenges which poses wide societal risks for the persons at risk. Stigmatisation and risk of employment were noted as areas of great concern if personal health information was shared – especially in the area of mental health. Risks were identified in sperm donors where biological parents were identified due to publicly available information. Fascinatingly, genomic risks pose long-line challenges as they not only pose a threat to the person and other living relatives, but also to those not yet born who are unable to provide consent. [13] Future discussions and policies should incorporate these considerations to foster public trust in genomic research.
Sample Size and Statistical Significance
Issues related to insufficient sample size, inappropriate level of statistical significance, and self-serving bias need to be addressed. Recommendations include incorporating effect size and confidence intervals, utilising appropriate sample sizes, and avoiding p-hacking and other self-serving biases.
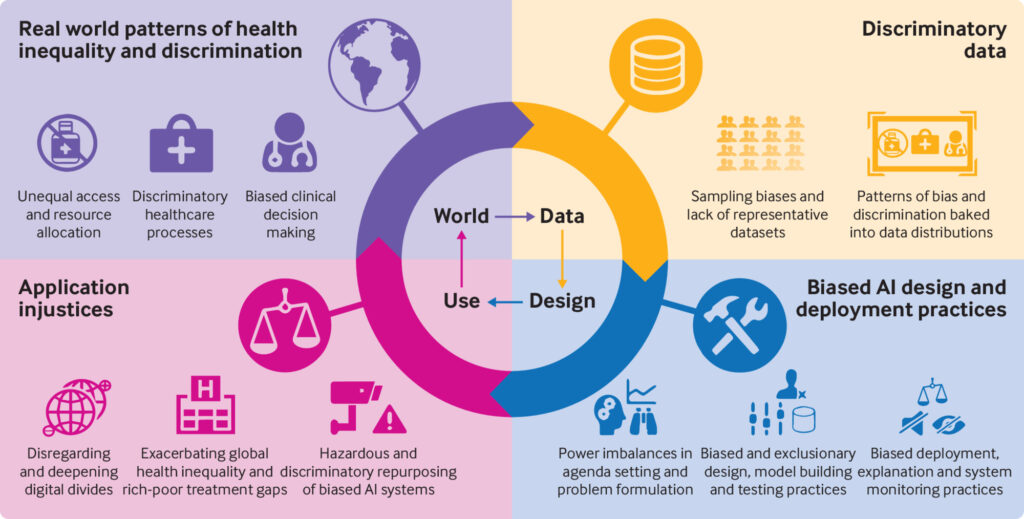
Introducing the SWOT-AI Framework
In using the aspects for consideration, a framework could be suggested when reviewing an AI tool. How this would be performed or framed is difficult, but AI deployment would form part of a strategic planning exercise. When trying to understand the competitive landscape, businesses could employ tools, such as a Strengths, Weaknesses, Opportunities, and Threats (SWOT) analysis.
The SWOT analysis – while fairly simple in its design – is a low-cost exercise that can accommodate a comprehensive data set when reviewing the competitive landscape. The outcome of this analysis is to understand the improvements that need to be made while also ensuring the optimisation of processes and operations when taking into account the various regulations. An important outcome of this analysis is also geared toward risk limitation. To this end, it could stand to reason that an internal AI SWOT analysis be performed by reviewing the designed tool. This tool could be used in line with the World Health Organization and The Lancet Commission’s best practices for AI. They include six core principles: protecting autonomy, promoting human well-being, ensuring transparency, fostering responsibility, ensuring inclusiveness, and promoting responsive and sustainable AI.
SWOT-AI Framework for Navigating AI in Healthcare Deployment
Strengths | Weaknesses |
Ensuring fairness and transparency. The product is human centred and designed for humanity. | It does not control inherent risks to privacy or security. |
Ethical AI The incorporation of ethical principles can enhance the system’s trustworthiness and societal acceptance. | Algorithmic Bias The deployment does not address relevant biases which can lead to unfair and discriminatory outcomes. |
Explainable AI There is well-defined transparency in the AI decision-making process. This also improves user understanding and trust. | Sponsorship Bias The deployment is biased towards the needs and outcomes of the funding source. This may lead to skewed AI research and development. |
Health Equity The deployment promotes fairness in healthcare and can lead to improved access and outcomes. | Data Privacy There is no clearly defined scope between data access and privacy protection. While this is a challenging area, poor data privacy is a great weakness. |
Sample Size Issues Inadequate testing data can limit the AI system’s effectiveness and generalisability. | |
Opportunities | Threats |
It enhances decision-making and is explainable, providing autonomy | Poor risk control, data could be harmful |
Ethical AI The deployment presents a unique opportunity to set industry standards and gain a competitive edge through ethical AI. | Algorithmic Bias The deployment poses legal and reputational risks due to biased decision-making. |
Explainable AI The deployment will fare better in user acceptance and compliance with regulations. | Sponsorship Bias The deployment poses a risk of conflicts of interest and skewed AI development. |
Health Equity The deployment would be able to reduce healthcare disparities and improve public health. | Data Privacy The deployment has a potential for breaches and regulatory non-compliance. |
Sample Size Issues The deployment shows inaccurate or biased conclusions in research and healthcare applications. |
The above analysis is just the start of the evaluation process for AI tools. The outcomes of which can continue to be scrutinised through this lens up until weaknesses and threats (ultimately risks) have been sufficiently managed and de-risked. The outcomes of the SWOT-AI process could also suggest the value framework of the tool to be developed and deployed.
Conclusion
Artificial Intelligence (AI) holds significant potential to revolutionise healthcare. It still requires careful consideration of ethical AI, explainable AI, health equity and algorithmic biases, sponsorship bias in medical literature, data privacy, genomics and privacy protection, and issues related to sample size and statistical significance. Any bias must be stated upfront and ensure that it’s not just a one-sided value proposition but more a statement based on the end users’ needs. Bias must be removed from the data set which would have a great effect on outcomes and accessibility. Gaps in data need to be addressed to limit errors and ensure safety.
A matrix analysis using the SWOT framework is suggested to provide an initial check on whether the designed intention of the tool would actually meet its specifications while ensuring high adherence to ethics. A standardised and transparent process would strengthen the tools and their algorithms being developed. It also ensures that the appropriate governance procedures are being followed.
References
- Sergei Polevikov, Advancing AI in healthcare: A comprehensive review of best practices, Clinica Chimica Acta, Volume 548, 2023, 117519, ISSN 0009-8981, https://doi.org/10.1016/j.cca.2023.117519. (https://www.sciencedirect.com/science/article/pii/S0009898123003212)
- Leslie D, Mazumder A, Peppin A, Wolters MK, Hagerty A. Does “AI” stand for augmenting inequality in the era of covid-19 healthcare?. BMJ. 2021;372:n304. doi:https://doi.org/10.1136/bmj.n304
- Hagendorff, T. Blind spots in AI ethics. AI Ethics 2, 851–867 (2022). https://doi.org/10.1007/s43681-021-00122-8
- Farhud DD, Zokaei S. Ethical Issues of Artificial Intelligence in Medicine and Healthcare. Iranian Journal of Public Health. 2021;50(11). doi:https://doi.org/10.18502/ijph.v50i11.7600
- Hui Wen Loh, Chui Ping Ooi, Silvia Seoni, Prabal Datta Barua, Filippo Molinari, U Rajendra Acharya, Application of explainable artificial intelligence for healthcare: A systematic review of the last decade (2011–2022), Computer Methods and Programs in Biomedicine, Volume 226, 2022, 107161, ISSN 0169-2607, https://doi.org/10.1016/j.cmpb.2022.107161. (https://www.sciencedirect.com/science/article/pii/S0169260722005429)
- Grother P, Ngan M, Hanaoka K. Face recognition vendor test part 3. Face Recognition Vendor Test (FRVT) Part 3: Demographic Effects. Published online December 2019. doi:https://doi.org/10.6028/nist.ir.8280
- Wilson, B., Hoffman, J., & Morgenstern, J. (2019). Predictive Inequity in Object Detection. ArXiv, abs/1902.11097.
- Brown TB, Mann B, Ryder N, et al. Language Models are Few-Shot Learners. arxivorg. Published online May 28, 2020. https://arxiv.org/abs/2005.14165
- UNESCO. I’d blush if I could: closing gender divides in digital skills through education. Published online January 1, 2019. doi:https://doi.org/10.54675/rapc9356
- Jefferson T. Sponsorship bias in clinical trials: growing menace or dawning realisation? Journal of the Royal Society of Medicine. 2020;113(4):148-157. doi:https://doi.org/10.1177/0141076820914242
- Nazish Khalid, Adnan Qayyum, Muhammad Bilal, Ala Al-Fuqaha, Junaid Qadir, Privacy-preserving artificial intelligence in healthcare: Techniques and applications, Computers in Biology and Medicine, Volume 158, 2023, 106848, ISSN 0010-4825, https://doi.org/10.1016/j.compbiomed.2023.106848. (https://www.sciencedirect.com/science/article/pii/S001048252300313X)
- Petrosyan A. Topic: Healthcare and cyber security in the U.S. Statista. Published October 18, 2022. https://www.statista.com/topics/8795/healthcare-and-cyber-security-in-the-us/#topicOverview
- Oestreich M, Chen D, Schultze JL, Fritz M, Becker M. Privacy considerations for sharing genomics data. EXCLI Journal. 2021;20:1243-1260. doi:https://doi.org/10.17179/excli2021-4002
Table of Contents