Introduction
The systematic scoping review on the use of artificial intelligence (AI) in healthcare systems in low- and middle-income countries (LMICs) reveals a range of findings and insights. AI has been proposed as a means to strengthen healthcare systems in these regions, showing promise in various applications like clinical decision support systems, treatment planning, triage assistants, and health chatbots. Moreover, the integration of AI technologies into existing healthcare systems requires careful planning and collaboration among healthcare providers, policy makers, and AI developers.
In this article, we will unpack the use of AI in healthcare systems in LMICs. We will explore three key themes: access, efficiency, and reliability. Through stakeholder perspectives and a comprehensive discussion, we will examine the potential benefits and challenges of integrating AI technologies into healthcare systems in LMICs. By the end of this article, readers will gain a deeper understanding of the implications of AI in improving healthcare outcomes in these regions. This article sums up a thematic glance into AI usage.
Improving Access to Healthcare through AI use in LMICs
Access to healthcare is a critical theme in the context of using AI in LMICs [1]. The implementation of AI in these healthcare systems has the potential to bridge gaps in medical services, especially in remote or underserved areas. AI can facilitate access to medical expertise through tools like diagnostic algorithms, telemedicine platforms, and health chatbots, potentially reaching rural and isolated populations who lack access to medical professionals.However, challenges remain, such as ensuring that AI-driven solutions are accessible to all segments of the population, including those with limited technology access or literacy.
Moreover, AI recommendations might suggest treatments that are not locally accessible or affordable, highlighting the need for AI tools to be tailored to the specific healthcare contexts and resources of LMICs [2] .The implementation of AI in LMICs has the potential to improve access to healthcare services, particularly in remote or underserved areas. However, challenges such as limited technology access and affordability of treatments need to be addressed to ensure that AI-driven solutions are accessible and beneficial to all segments of the population in LMICs. Tailoring AI tools to the specific healthcare contexts and resources of LMICs is crucial for their successful integration into these healthcare systems. One of the core aspects of this thematic glance into AI usage provides an oversight of how important access is.
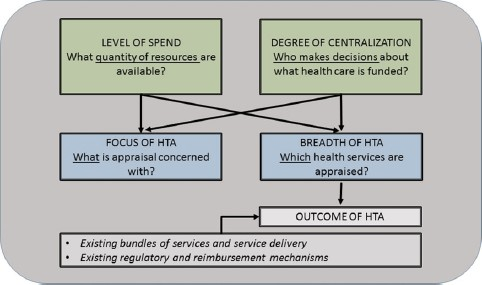
Enhancing Healthcare Efficiency with AI Usage
Efficiency in healthcare systems can be significantly enhanced through the use of AI, as observed in LMICs. AI technologies have shown promise in improving workflows, reducing time to treatment and diagnosis, and assisting healthcare workers in managing their workloads more effectively. For instance, AI-based ultrasound triage allows non-specialist healthcare workers to perform screenings, thereby reducing the workload of sonographers. Automated treatment planning tools also decrease the time oncologists spend on creating treatment plans. These efficiencies can be especially beneficial in LMICs, where healthcare resources are often stretched thin.
However, the integration of AI into healthcare practices must be done cautiously, as there are reports of increased workloads in settings where there is low capacity for adopting new AI tools, indicating that efficiency gains are not universal and depend on the specific implementation and context [3]. AI-based tools such as ultrasound triage and automated treatment planning can reduce the workload of healthcare workers and improve patient outcomes. However, careful consideration must be given to the implementation and context of AI in order to ensure that efficiency gains are realized and that the benefits are universal. Efficiency is also an important avenue within the thematic glance into AI usage.
Ensuring the Reliability of AI in Healthcare
Reliability is a crucial concern when integrating AI into healthcare systems, particularly in LMICs. The effectiveness and trustworthiness of AI tools depend on their ability to provide accurate and contextually appropriate recommendations. Studies have shown varying degrees of concordance between AI tools and physician decisions. In some cases, AI demonstrated high agreement with physicians, while in others, there were significant discrepancies, often due to the AI’s training on data from different contexts or its failure to consider local disease incidence and treatment options[4] . This inconsistency raises concerns about the reliability of AI in making clinical decisions.
Ensuring the reliability of AI in healthcare requires rigorous testing and validation of these tools in the specific environments where they will be used, along with continuous monitoring and improvement to adapt to changing healthcare needs and contexts. The reliability of AI in healthcare systems, especially in LMICs, is a critical factor to consider. Studies have shown that while AI tools can demonstrate high agreement with physicians in some cases, there can also be significant discrepancies due to training on different data contexts or failure to consider local disease incidence and treatment options. To ensure the reliability of AI, rigorous testing, validation, and continuous monitoring and improvement are necessary to adapt to changing healthcare needs and contexts. Another element within the thematic glance into AI usage is the scope of reliability.
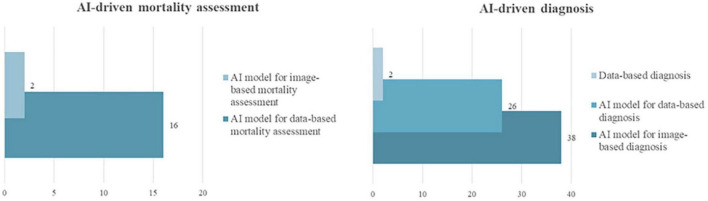
Stakeholder Perspectives: AI Use in LMICs
The deployment of AI in healthcare within LMICs elicits mixed reactions among social partners and stakeholders, reflecting the complex interplay of advancement and challenges in these settings. For healthcare professionals, AI presents a promising avenue to alleviate burdensome workloads, enhance diagnostic precision, and streamline treatment processes. Yet, there exists an underlying apprehension about the potential erosion of clinical skills, the misalignment of AI recommendations with local healthcare realities, and the hurdles in integrating these technologies into existing infrastructures. These concerns are amplified by the often-limited resources and varying levels of digital literacy among healthcare workers in LMICs, raising questions about the practicality and inclusiveness of AI applications in these regions [5].
From the perspective of patients and the wider community in LMICs, AI in healthcare is often viewed through the lens of accessibility and equity. There’s an optimistic outlook towards the potential of AI to democratize healthcare access, particularly in remote or underserved areas. However, this optimism is tempered by apprehensions about the affordability of AI-driven healthcare solutions, their cultural appropriateness, and the potential for increased healthcare disparities [6]. The involvement of international agencies and private entities in AI development and deployment further complicates the landscape, as it could lead to solutions that are misaligned with local needs and contexts. The engagement of local stakeholders in the development and implementation of AI solutions is thus crucial to ensure that these technologies are tailored to the unique challenges and needs of LMICs, fostering equitable and sustainable healthcare advancements.
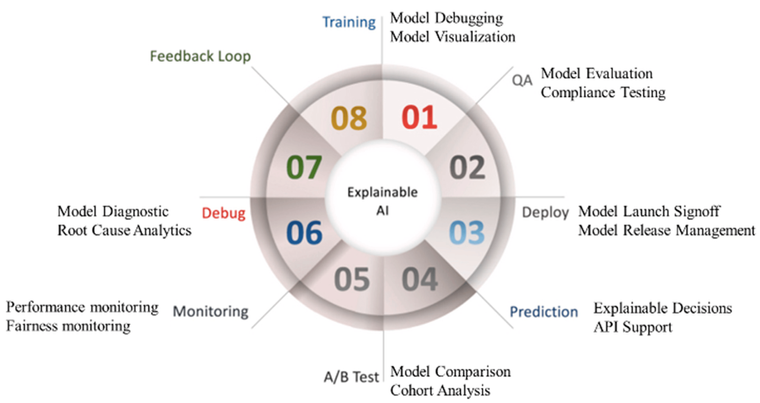
The Impact and Challenges of AI in Healthcare
One of the key observations is the mixed impact of AI on healthcare workflows and the time taken for treatment and diagnosis. AI technologies have been found to improve workflows in certain scenarios, such as enabling non-sonographer healthcare workers to perform AI-based ultrasound triage, which reduces the workload of formally trained sonographers. Automated radiation treatment planning has similarly reduced the time spent by oncologists in creating treatment plans.
However, there are also reports of increased workloads in clinical settings with limited capacity to adopt new AI tools [7]. A thematic glance into AI usage would be necessary to unpack which areas to focus on improvement. Regarding the reliability of AI tools, there is a notable variance in the concordance between AI and physician decisions. Some AI tools showed high agreement with physicians in certain applications, while others exhibited discordance, particularly in cases where AI recommendations did not align with local treatment options or contexts.
Examples on the Impact and Challenges of AI in Healthcare
For instance, the IBM Watson for Oncology and Baidu Inc’s ‘Brilliant Doctor’ system showed varying degrees of agreement with physicians’ clinical decisions, with discrepancies often arising due to the AI’s training on data from different contexts or its failure to account for local disease incidence and treatment options [8].User-friendliness and compatibility with existing infrastructure are also critical. There were instances where AI tools required excessive input from physicians, lacked integration with existing IT systems, or faced issues like app crashes and mistranslations. This highlights the importance of ensuring that AI technologies are adapted to the local context and are easy to use by healthcare professionals [9].
Issues such as excessive input requirements, lack of integration with IT systems, and technical glitches can hinder their effectiveness. Therefore, it is essential to adapt AI technologies to the local context and ensure they are easy to use for healthcare professionals. The use of AI in silos by international agencies in LMICs could further fragment health systems. Over-reliance on AI might lead to erosion of clinical and critical thinking skills, and there’s the risk of AI tools recommending treatments that are not locally accessible or affordable [10]. The use of AI in sensitive areas such as mental health could raise ethical concerns, especially in contexts where technology access is controlled or where AI applications might lead to stigmatization.
Concluding Remarks on AI Use in LMICs
Collaboration between technology developers, healthcare professionals, and local communities is crucial to ensure that AI solutions are not only technologically advanced but also culturally and contextually relevant to the specific needs of LMICs. By reviewing the three relevant themes of AI applicability in LMICs, it strengthens the understanding of how digital innovations can enhance health systems. This article underscores the nuances of AI tools and efficacy in structures where resources may be limited however should be balanced to take in account that ethical considerations. A multifaceted approach must be taken in implementing AI utilization in medical conditions but the properties of clinical AI are key merits in contemporary healthcare landscape.
References
- Ciecierski-Holmes, T., Singh, R., Axt, M. et al. Artificial intelligence for strengthening healthcare systems in low- and middle-income countries: a systematic scoping review. npj Digit. Med. 5, 162 (2022). https://doi.org/10.1038/s41746-022-00700-y
- Lambert, S.I., Madi, M., Sopka, S. et al. An integrative review on the acceptance of artificial intelligence among healthcare professionals in hospitals. npj Digit. Med. 6, 111 (2023). https://doi.org/10.1038/s41746-023-00852-5
- López DM, Rico-Olarte C, Blobel B, Hullin C. Challenges and solutions for transforming health ecosystems in low- and middle-income countries through artificial intelligence. Front Med (Lausanne). 2022 Dec 2;9:958097. doi: 10.3389/fmed.2022.958097. PMID: 36530888; PMCID: PMC9755337.
- Alami, H., Rivard, L., Lehoux, P. et al. Artificial intelligence in health care: laying the Foundation for Responsible, sustainable, and inclusive innovation in low- and middle-income countries. Global Health 16, 52 (2020). https://doi.org/10.1186/s12992-020-00584-1
- Khanna, Narendra N., Mahesh A. Maindarkar, Vijay Viswanathan, Jose Fernandes E Fernandes, Sudip Paul, Mrinalini Bhagawati, Puneet Ahluwalia, Zoltan Ruzsa, Aditya Sharma, Raghu Kolluri, and et al. 2022. “Economics of Artificial Intelligence in Healthcare: Diagnosis vs. Treatment” Healthcare 10, no. 12: 2493. https://doi.org/10.3390/healthcare10122493
- Morrow E, Zidaru T, Ross F, Mason C, Patel KD, Ream M, Stockley R. Artificial intelligence technologies and compassion in healthcare: A systematic scoping review. Front Psychol. 2023 Jan 17;13:971044. doi: 10.3389/fpsyg.2022.971044. PMID: 36733854; PMCID: PMC9887144.
- Joseph B Babigumira, Alisa M Jenny, Rebecca Bartlein, Andy Stergachis, Louis P Garrison, Health technology assessment in low- and middle-income countries: a landscape assessment, Journal of Pharmaceutical Health Services Research, Volume 7, Issue 1, March 2016, Pages 37–42, https://doi.org/10.1111/jphs.12120
- Mills A. Reflections on the development of health economics in low- and middle-income countries. Proc Biol Sci. 2014 Aug 22;281(1789):20140451. doi: 10.1098/rspb.2014.0451. PMID: 25009059; PMCID: PMC4100502.
- Oduoye MO, Fatima E, Muzammil MA, Dave T, Irfan H, Fariha FNU, Marbell A, Ubechu SC, Scott GY, Elebesunu EE. Impacts of the advancement in artificial intelligence on laboratory medicine in low- and middle-income countries: Challenges and recommendations-A literature review. Health Sci Rep. 2024 Jan 4;7(1):e1794. doi: 10.1002/hsr2.1794. PMID: 38186931; PMCID: PMC10766873.
- Williams D, Hornung H, Nadimpalli A, Peery A. Deep Learning and its Application for Healthcare Delivery in Low and Middle Income Countries. Front Artif Intell. 2021 Apr 29;4:553987. doi: 10.3389/frai.2021.553987. PMID: 33997772; PMCID: PMC8117675.
Table of Contents