Introduction
The use of artificial intelligence (AI) in healthcare has rapidly grown due to its potential impact on healthcare delivery and efficiency. The digitization of health data alongside advancements in computing power and algorithms has made AI-supported healthcare increasingly more common. Healthcare is facing challenges such as digital health data exceeding the human capacity to process and analyze it, healthcare staff shortages, an aging population, and increasing costs. AI provides a solution to address these challenges by improving patient care through automated clinical workflows, interpretation of clinical findings, and prediction of health outcomes. However, the value and effectiveness of AI in clinical practice remain uncertain, and guidance on proper implementation is crucial. Reports on AI accuracy and precision are insufficient in demonstrating clinical effectiveness and adoption, and evidence supporting clinical effectiveness is limited. Despite its potential, the clinical applicability of AI in healthcare requires further investigation.
The utilization of health technology assessment (HTA) is crucial for stakeholders and decision makers to efficiently utilize health resources through the development of policies prior to their implementation in clinical practice. However, the absence of regulatory policies inhibits the integration of AI. Despite the numerous developed AIs, the lack of distinct guidelines poses a challenge for researchers, policy makers, and developers to identify suitable AIs for clinical adoption.
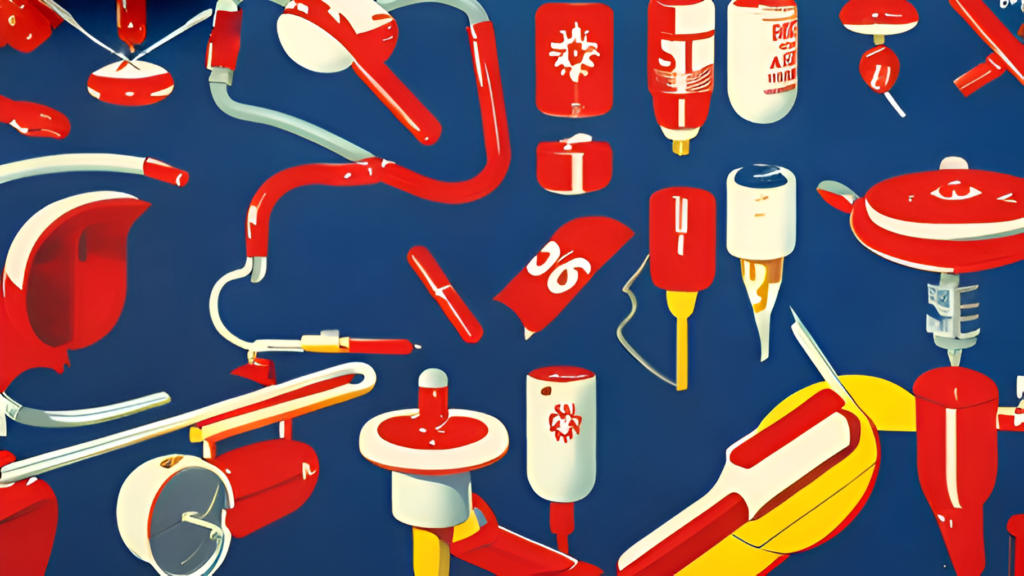
Real-World Challenges of Implementing Artificial Intelligence in Healthcare: Balancing Economic, Legal, and Ethical Implications
The roots of AI can be traced back to the 1950s, when British mathematician Alan Turing pondered whether machines could possess intelligence. While it took over a decade for AI to be introduced to life sciences, the 1970s marked its expansion into healthcare. The 1980s saw AI’s integration into more clinical settings, utilizing artificial neural networks, Bayesian networks, and hybrid intelligence systems. Examples currently seen include the use of AI as a diagnostic tool in cancer, treatment tool in cardiology, machine learning, natural language processing as well as robotics.
Although the use of AI in healthcare is gaining traction, it remains largely limited. The U.S. Food and Drug Administration has only approved approximately 20 to 25 AI applications for healthcare, most of which are targeted towards consumers and focus on low-risk conditions like screening for eye disease or detecting specific heart-rhythm issues through an Apple Watch. There are currently only a few AI applications being implemented in hospitals for patient care, but the situation is slowly changing.
Some key challenges posed by AI in the healthcare environment include technology in a real-world context, clinical dimension, human and cognitive dimensions (patients and clinicians), professional and organizational dimensions, economic as well as legal and ethical aspects.
Economic challenges for AI in healthcare
Adapting AI to a local environment requires significant investments and expenditures, including continuous performance and quality tests, infrastructure upgrades, human expertise, and training. However, many healthcare organizations lack the necessary resources to collect the data needed to train and adapt AI to their local population and clinical environment.
In health systems that use activity-based financing, some clinicians may prioritize higher-paying codes for each clinical activity, leading to biased data. AI applications trained on this data could amplify biases that do not reflect actual clinical conditions, resulting in harm to patients, clinicians, organizations, and health systems. Additionally, some AI may be too cautious, resulting in unnecessary testing and treatment and increased costs.
Legal and ethical aspects and considerations
The increasing use of AI in clinical decision-making poses several challenges, primarily in determining legal responsibility for errors or malfunctions. If the clinical decision is based on an erroneous clinical recommendation from the AI, then who will be held responsible? Is it the technology developer, technology provider, clinician, organization, or do they all share responsibility, and how? The distinction between AI as a decision-making support tool and a decision-making tool is important in defining responsibility. Access to patient data from different organizations raises concerns about privacy and consent. Regulators need to determine ownership and responsibility for data collection, use, and transmission. The ethical implications of AI affect all dimensions of healthcare.
Addressing Challenges and Embracing Opportunities in the Use of AI in Healthcare
ISPOR held a conference and discussed how big data and AI can help improve healthcare decision making with the below listed speakers:
- Marc Boutin, JD; National Health Council; Washington, DC, USA,
- Rachael Callcut, MD, MSPH; University of California, San Francisco Medical Center, Center for Digital Health Innovation; San Francisco, CA, USA, and
- Nigam Shah, MBBS, PhD; Stanford University; Stanford, CA, USA.
Some of the key points from the discussions:
The next big thing in health economics and outcomes research is AI
Overall, artificial intelligence in healthcare can be a powerful tool in improving systems and addressing the challenges facing the industry. However, it must be used in a way that prioritizes patient outcomes and takes into account the broader determinants of health. As healthcare delivery continues to evolve, there will likely be even greater opportunities for AI to drive innovation and change.
The potential of AI in healthcare exists for better care and outcomes, especially in personalized health, telemedicine, and digital medicine. The pandemic has accelerated the adoption of remote healthcare delivery, leading to a hybrid model which combines remote and in-person care. The need for health technology to focus on patient outcomes rather than profits and incorporate the broader determinants of health. Overall, the speakers believed that AI could help address the challenges facing the healthcare industry and drive positive change.
The discussions emphasized the importance of looking at wellness holistically, incorporating all determinants of health, including social environment and economic factors. They see great potential in using augmented intelligence and behavioral science to personalize health for individuals. AI can also be used to read through the noise of the overwhelming amount of information out there, and to drive better care and outcomes.
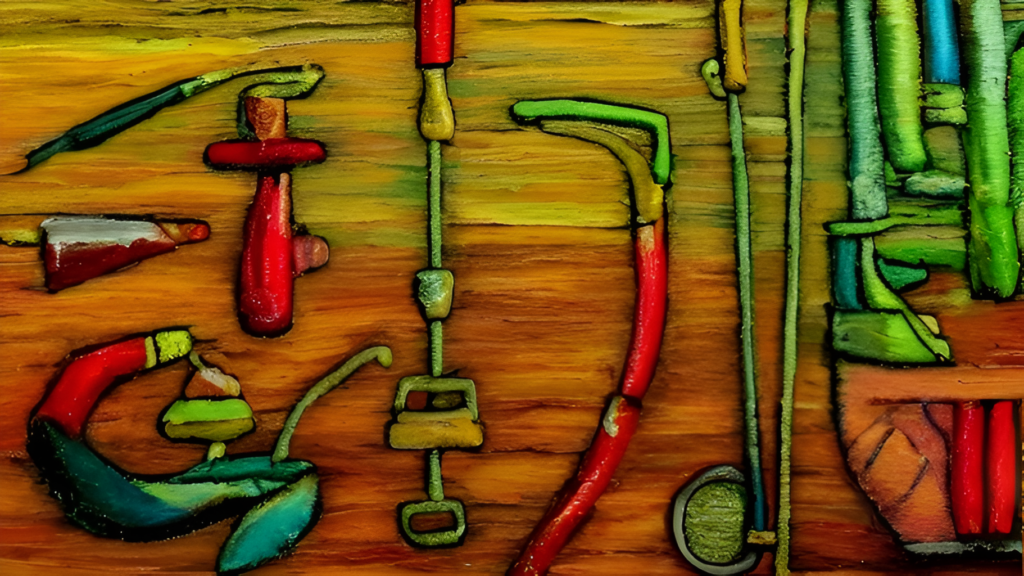
Can artificial intelligence in healthcare be used to reduce the cost of care in the United States?
There are various ways in which AI can be used in healthcare, including chronic disease management, remote monitoring, and population health management. They highlight how AI can improve patient outcomes, reduce costs, and drive positive change. However, there are concerns around bias in AI and the need to focus on patient outcomes rather than profits.
AI could help in chronic disease management by identifying patients who would benefit from expensive care and providing an early warning system for potential complications or side effects. This could help patients understand when to seek medical care and lead to more efficient use of healthcare resources. AI could also help move expensive care provided in hospital settings to lower-cost outpatient environments, leveraging remote monitoring devices and medical-grade information.
Population health management is another area where AI can be useful. By using AI to identify potential gaps in care and drilling down on population-level data to understand community health profiles, healthcare systems can develop intervention programs to improve patient outcomes and reduce healthcare costs. Preventative care is important and the ability to predict and prevent negative health outcomes before they occur.
Finally, bias typically resides in the data rather than the algorithms themselves and highlight the difference between systematic errors in the algorithm’s output versus systematic differences in the accrual of benefit. It is important to focus on the latter in order to ensure fair and equitable healthcare access and outcomes for all patients.
Is this cost effective?
Overall, the potential of artificial intelligence in healthcare is enormous, but it must be used carefully and strategically to ensure that it aligns with the needs and desires of patients, which may not always align with the interests of the healthcare industry. While there are opportunities for AI to address the inefficiencies in the healthcare system and streamline aspects of care, we must also consider the indirect costs to patients, such as travel and time, which are often not factored into algorithms that capture costs, when evaluating the true cost-effectiveness of AI in healthcare. Regulatory challenges must be overcome, but as the conversation shifts from “if and whether” to “how” to use AI effectively, we can start to unlock the full potential of this technology to improve patient outcomes and deliver more personalized care.
The COVID-19 pandemic has accelerated the adoption of telemedicine and digital medicine, leading to a shift in the way healthcare is delivered. As a result, the use of technology in healthcare is likely to remain as a hybrid model. AI also has the potential to improve the delivery of care, especially on the diagnostic side. However, it is important to ensure that AI technology is aligned with the needs and desires of patients and to make sure that it is cost-effective.
The effect on health economics
The effect on health economics of the integration of AI in healthcare is significant. Health economists play a crucial role in ensuring that the utilization of AI in healthcare is economically viable, just like any other health intervention. They need to proactively design economic incentive systems that promote the use of AI in healthcare and ensure that it provides better care while still being cost-effective. Health economists must also claim their stake in the deployment and validation of AI in healthcare to avoid leaving the field predominantly driven by technologists and doctors. The analysis of the downstream impact of AI on healthcare and the measurement of its economic impact and outcomes are fundamental tasks that health economists need to undertake. By doing so, they can better measure the return on investment and ensure that AI is utilized effectively in healthcare.
Key takeaways
- The use of AI in healthcare is growing rapidly and has the potential to improve patient care and outcomes by addressing challenges such as healthcare staff shortages, an aging population, and increasing costs.
- The value and effectiveness of AI in clinical practice remain uncertain, and proper guidance on implementation is crucial.
- Regulatory policies are needed to enable the integration of AI in healthcare, and the absence of distinct guidelines poses a challenge for researchers, policy makers, and developers to identify suitable AIs for clinical adoption.
- AI applications in healthcare face economic challenges such as significant investments, biased data, and increased costs due to unnecessary testing and treatment.
- Legal and ethical aspects need to be considered when using AI in clinical decision-making, including determining legal responsibility for errors or malfunctions and ensuring privacy and consent for patient data.
References
- Voets MM, Veltman J, Slump CH, Siesling S, Koffijberg H. Systematic Review of Health Economic Evaluations Focused on Artificial Intelligence in Healthcare: The Tortoise and the Cheetah. Value Health. 2022 Mar 1;25(3):340–9.
- TWinFM. Everything You Need to Know About AI in Healthcare [Internet]. TWinFM. [cited 2023 Mar 28]. Available from: https://www.twinfm.com/article/everything-you-need-to-know-about-ai-in-healthcare
- 7 Life-Saving AI Use Cases in Healthcare [Internet]. [cited 2023 Mar 28]. Available from: https://www.v7labs.com/blog/ai-in-healthcare
- AI Will Give Your Doctor Superpowers | UCSF Magazine [Internet]. 2023 [cited 2023 Mar 28]. Available from: https://magazine.ucsf.edu/ai-will-give-your-doctor-superpowers
- Alami H, Lehoux P, Auclair Y, de Guise M, Gagnon MP, Shaw J, et al. Artificial Intelligence and Health Technology Assessment: Anticipating a New Level of Complexity. J Med Internet Res. 2020 Jul 7;22(7):e17707.
- How Big Data and Artificial Intelligence Can Help Improve Healthcare Decision Making [Internet]. ISPOR | International Society For Pharmacoeconomics and Outcomes Research. [cited 2023 Mar 28].
Table of Contents